Years ago, the editor of a prominent U.S. agricultural journal showed me a manuscript he intended to reject solely because it focused on applications of "artificial neural networks" in agriculture. He expressed weariness at the influx of such papers, particularly from researchers in developing countries. At that time, machine learning research was virtually nonexistent within our department. Ironically, I recently received a manuscript on the same topic for review, co-authored by the same individual.
Today, a significant portion of the research papers I review in agriculture focus on the applications of machine or deep learning. However, a notable shift has emerged: a substantial increase in submissions from computer scientists or electrical engineers. While I acknowledge their enthusiasm, I often find these papers lack a deep understanding of the unique challenges and complexities of the agricultural domain. It's perplexing to me how researchers with primary expertise in programming and machine learning can assume they possess a comprehensive grasp of the intricacies of agriculture.
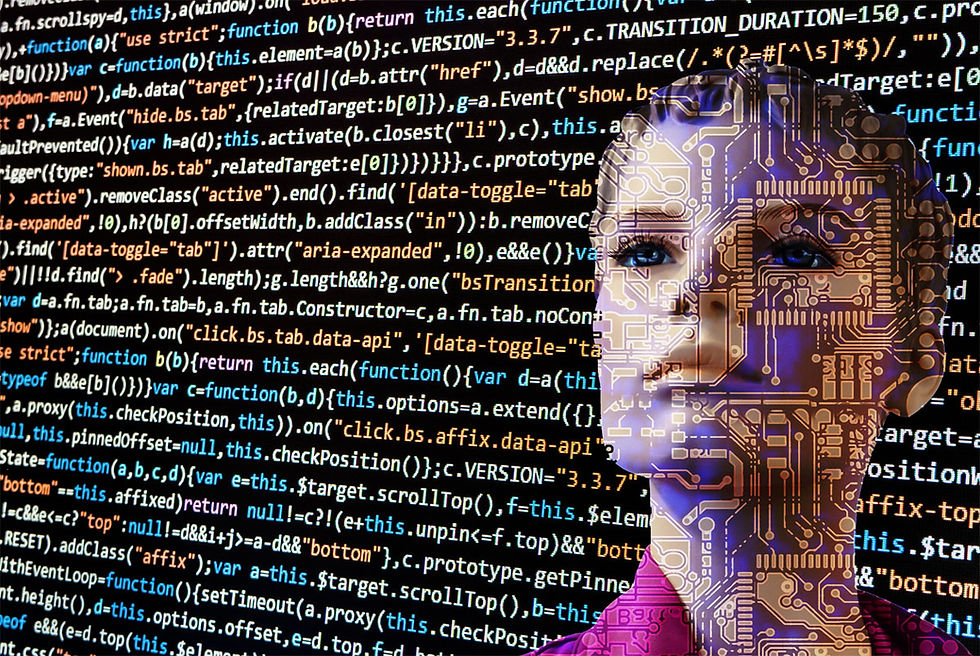
This trend extends beyond academia. There's a growing tendency to prioritize computer science expertise in agricultural research, potentially at the expense of valuable domain knowledge. Agricultural and biological engineering departments increasingly seek to hire graduates with strong computer science backgrounds, recognizing the importance of AI. Simultaneously, leading technology companies like Amazon and Facebook, as well as major financial institutions, are actively recruiting agricultural and biosystems engineers for data science roles, leveraging their domain expertise for applications such as fraud detection. These companies often offer significantly higher salaries than those available within the agricultural sector, leading to a significant brain drain as talented individuals leave the field. I personally know of at least two agricultural engineering PhD graduates currently employed by banks, and another who now applies their expertise in medical imaging.
From a purely economic perspective, it's understandable why a biosystems engineer with expertise in computer vision and AI might be drawn to other sectors. The agricultural industry often struggles to compete with the lucrative salaries offered by tech giants and financial institutions for individuals with these highly sought-after skills.
A common adage among AI experts emphasizes that "AI is only half the solution." This highlights the critical role of data and the hardware required for data collection. Tech giants like Google and Facebook have amassed vast datasets over years, forming the foundation for their sophisticated AI algorithms. In stark contrast, only a small fraction of U.S. agriculture (estimated at 11%) utilizes sensor technologies.
Many farmers remain content with traditional, technology-agnostic approaches to water, nutrient, pest, and disease management. This significant data gap poses a major challenge for AI adoption in agriculture. While publicly available datasets exist, they often lack the specific characteristics and granularity required for meaningful agricultural applications. In the absence of "transfer learning" – the ability to adapt existing models to new domains – researchers must invest substantial time and resources in collecting and labeling large, high-quality datasets to develop accurate and effective AI models.
Given the current state of AI technology, it's crucial to question whether IoT-enabled wireless sensor networks, advanced imaging systems, and sophisticated AI models can truly replace the invaluable intuition and experience of farmers.
A significant concern arises from the unrealistic expectations surrounding AI in agriculture. Many, particularly those without deep AI expertise, anticipate magical outcomes while relying on unreliable sensors or attempting to integrate logically incompatible sensor data. This often leads to an "AI-centric" approach, where careful observation and critical analysis are replaced by a "black-box" mentality – simply "throwing everything into the algorithm" and expecting miraculous results. This misplaced faith in AI as a superior, almost mystical entity can hinder progress and lead to misguided decision-making.
Before fully embracing AI as a reliable solution in agriculture, we must prioritize the development of robust infrastructure. This necessitates addressing critical challenges such as:
Technology adoption by growers: Fostering widespread acceptance and integration of AI-driven technologies among the farming community.
Sensor accuracy and reliability: Ensuring the consistent and accurate collection of high-quality data through reliable and robust sensor technologies.
Data collection in remote areas: Overcoming the logistical and technological hurdles of collecting data from geographically dispersed and often remote agricultural sites.
Connectivity and data transfer: Establishing reliable and high-speed internet connectivity to facilitate seamless data transfer and real-time AI analysis.
Addressing critical measurement gaps: Developing and deploying novel sensors to measure crucial environmental parameters that are currently not adequately monitored.
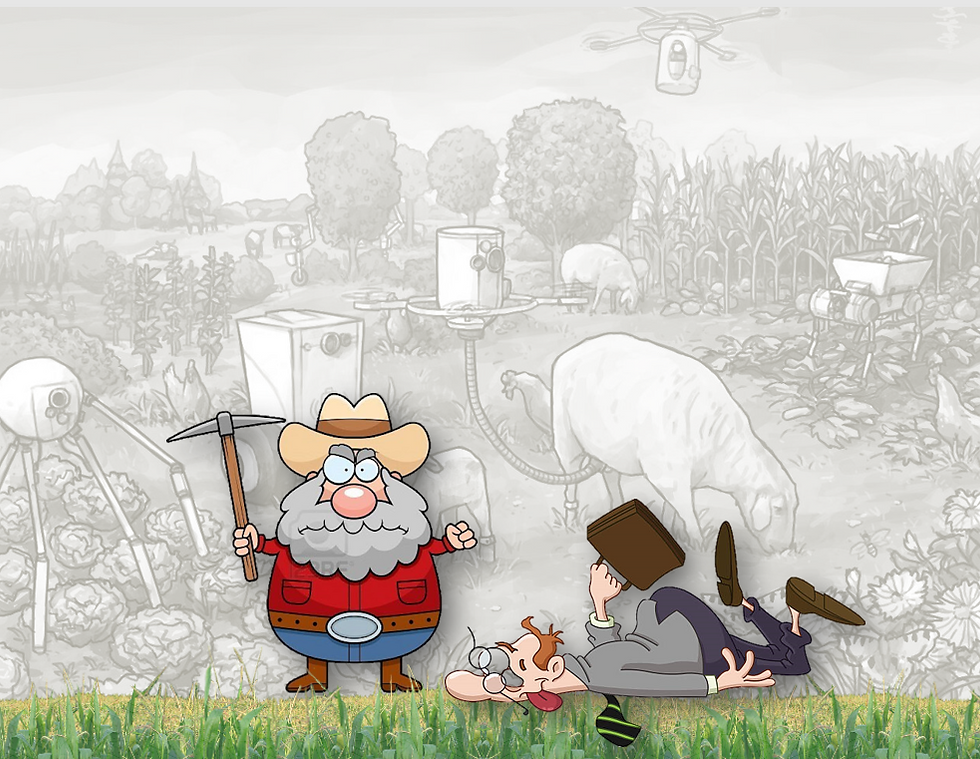
Given our decades-long struggle to effectively address fundamental challenges like water scarcity in agriculture using established methodologies, it's difficult to envision how "black-box" AI models, with their inherent opacity, can provide viable solutions.
Considering the existing reluctance among many growers to invest in basic sensor technologies, it seems unlikely that we can readily convince them to adopt the more complex and expensive infrastructure required for AI-driven solutions, which necessitates significant investments in sensors, hardware, software, and connectivity.
I am deeply concerned about the nature of agricultural engineering education in the current AI-driven landscape. Students may spend a significant portion of their education immersed in computer-based tasks such as image annotation and coding, potentially neglecting the crucial hands-on experience that is fundamental to understanding the complexities of agricultural systems.
Agriculture, unlike many other disciplines, demands a deep understanding of real-world field conditions, requiring students to gain practical experience through hands-on engagement with the agricultural environment. Have we reached a point where we believe we have fully grasped the intricacies of agriculture, to the extent that we can now rely solely on simulated or virtualized learning experiences?
I am concerned that within the next five to ten years, we may face a critical realization: that addressing the multifaceted challenges of agriculture will necessitate a more holistic approach than simply relying on "semantic image segmentation" techniques. By that time, however, the damage to the field may already be irreversible due to the current trajectory of AI research and implementation in agriculture.
In my opinion, several critical issues are contributing to this concerning trajectory:
Misaligned Research Priorities: In many cases, research efforts in AI for agriculture appear driven by the pursuit of funding, publications, and academic advancement rather than by a genuine desire to solve pressing agricultural problems.
Talent Drain and Expertise Gap: The agricultural sector is experiencing a significant loss of talented individuals to other industries, particularly those offering higher salaries in fields like technology and finance. Simultaneously, there's a growing reliance on non-experts in the field of agriculture, potentially leading to a critical gap in domain-specific knowledge.
Focus on Programming over Problem-Solving: Educational programs increasingly prioritize the development of advanced programming skills over the cultivation of critical thinking, problem-solving, and practical agricultural expertise among aspiring agricultural engineers.
Overreliance on AI as a Panacea: There's a prevailing tendency to treat AI as a magical solution to all agricultural challenges, overlooking the limitations of current technologies and the crucial need for a multi-faceted approach.
Neglect of Critical Infrastructure: Insufficient attention is being paid to the development of robust infrastructure, including reliable sensor networks, high-speed connectivity, and data management systems, which are essential for successful AI implementation in agriculture.
Here's parts of the best article I've read so far about "Artificial Intelligence". I strongly recommend it:
Dr. Melanie Mitchell, Davis Professor of Complexity at Santa Fe Institute
"... In 1892, the psychologist William James said of psychology at the time, “This is no science; it is only the hope of a science” [84]. This is a perfect characterization of today’s AI. Indeed, several researchers have made analogies between AI and the medieval practice of alchemy. In 1977, AI researcher Terry Winograd wrote, “In some ways [AI] is akin to medieval alchemy. We are at the stage of pouring together different combinations of substances and seeing what happens, not yet having developed satisfactory theories...but...it was the practical experience and curiosity of the alchemists which provided the wealth of data from which a scientific theory of chemistry could be developed” [85]. Four decades later, Eric Horvitz, director of Microsoft Research, concurred: “Right now, what we are doing is not a science but a kind of alchemy” [86]. In order to understand the nature of true progress in AI, and in particular, why it is harder than we think, we need to move from alchemy to developing a scientific understanding of intelligence. "
Comentários