In this article, I'm going to discuss the following topics:
Importance of measuring leaf wetness and temperature in agriculture
Leaf wetness sensor technologies
Temperature dependence and reliability of leaf wetness sensors
Wetness and temperature measurements using the research-grade INSHU LWS sensor
Temperature-compensation algorithm for the INSHU LWS sensor
Comparison between INSHU LWS and a well-known sensor
Why Is It Important to Measure Leaf Wetness and Temperature?
Air temperature, relative humidity, and surface wetness are microclimate variables commonly used for crop and fruit loss, and disease management. Knowing the relationship between weather and the epidemiology of pathogens, some scientists have tried to decrease pest infestations through modifying the microclimate around crops. Climate change influences on pest and pathogen dynamics are also known and extensively discussed in the literature.
Leaf [surface] wetness duration (LWD) is used for monitoring, prediction and management of diseases in high value crops. Leaf wetness duration is an important variable in plant disease epidemiology used to quantify pathogens exposure to free water. Research has shown that bacteria and fungi are closely related to the humidity and wetness of fruit and canopy, and can be used to determine critical times for chemical spray applications.
Fruit surface wetness and temperature are important parameters in pre- and post-harvest crop loss management. Some varieties of fresh-market fruit, such as sweet cherry, tomatoes and grapes are sensitive to the presence of moisture on fruit surface (i.e. fruit surface wetness), which may lead to fruit cracking/splitting and loss of fresh-market values.
For example, sweet cherry fruit cracking caused by rainwater is a major source of financial loss to growers around the globe and especially in the pacific region of the United States. Sweet cherry faces the challenge of crop loss up to 90% in some varieties due to fruit splitting/cracking from seasonal rains prior to harvest. Timely removal of rainwater from fruit surface requires accurate and real-time monitoring of fruit wetness in the field as part of a crop loss management system.
Surface wetness and temperature of diseased fruits may be different from that of healthy ones. Quantification of fruit surface wetness and temperature could thus provide a promising way for monitoring and prediction of disease/pests in different crops. This is why many disease warning systems rely on leaf wetness as an input to help determine critical times to spray crops against diseases.
Leaf Wetness Sensor Technologies
Due to small gradients in plant canopies, microclimate measurements within canopy require sensitive sensors. In recent years, a wide range of low-cost microclimate monitoring sensors have been developed, which can be used for the purpose of phenological modelling. In-field microclimate measurements can be carried out using mobile or stationary setups. The microclimate measurements can also be integrated into a wireless sensor network (WSN) to collect information on both spatial and temporal variations.
Leaf wetness can be due to dew, rainfall, or irrigation events. Surface wetness duration is often measured indirectly using in-field sensors. A leaf wetness sensor (LWS) measures either the resistance or dielectric constant of a printed grid of interlacing copper wires and relates it to the presence of water on the surface.
Dielectric vs resistance grid leaf wetness sensors
Although different studies have confirmed the impacts of painting on the sensitivity of the resistance grid leaf wetness sensors, they are often deployed unpainted because it is time-consuming to paint and may result in non-uniformity among the sensors. Currently, there is no standardized method for the calculation of leaf wetness onset and duration using resistance-type leaf wetness sensors.
Dielectric leaf wetness sensors are a more recent category and detect the presence of water or ice on the surface of the sensor based on their much higher dielectric constants (80 and 5, relatively) compared to that of air (1). Unlike the resistant-type sensors, dielectric leaf wetness sensors come with a surface coating that does not absorb water. This allows for a much higher sensor sensor-to-sensor uniformity.
Cylindrical leaf wetness sensors
Almost all commercial leaf wetness sensors fall into the category of flat plate sensors that mimic plant leaves. Besides the flat plate sensors, cylindrical type leaf wetness sensors have also been developed for non-commercial use. Sentelhas et al. (2007) compared these two types of sensors in four different environments. The overall results showed that the cylindrical sensor overestimated leaf wetness duration in a more humid climate and detected wetness earlier than flat-type sensors. Several studies have compared physical and empirical models with visual observations and in-field sensors.
Detection of leaf wetness and temperature using thermal-RGB imagery
In an experiment, my team and I at the university investigated the feasibility of thermal-RGB imaging for detecting cherry fruit surface wetness level and duration (Osroosh and Peters, 2019). We carried out an experiment in plots of cherry varieties at the Roza Farm of Washington State University. We used a rain simulator to wet cherry fruits and leaves. The in-field sensing setup included two custom-built thermal-RGB imagers, a microclimate-measuring unit and two leaf wetness sensors. We developed a computer vision algorithm to identify leaves and cherry fruits in thermal and RGB images and extract their surface temperatures (Fig. 2). We were able to relate the surface temperature changes to wetness.
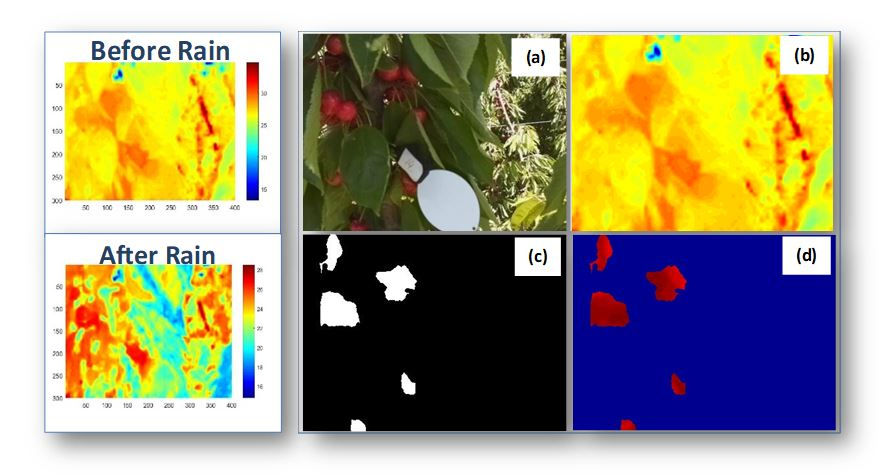
Temperature Dependence and Reliability of Leaf Wetness Sensors
There are many debates on the reliability of leaf wetness sensors, especially the resistance grid type, as an important input to many pest and disease forecast models. One of the major issues ignored by leaf wetness sensor manufactures is the so called "temperature dependence" of leaf wetness sensors. This makes them unreliable for automation projects. This issue is also seen in many other environmental sensors such as soil moisture and CO2 sensors used in indoor and outdoor agriculture (Blog article: Why Soil Temperature Fluctuations Result in Inaccurate Soil Moisture Measurements?).
The temperature dependence means that sensor output changes not only as a result of water or ice presence on the surface of the sensor, but also due to a change in air temperature. It is interesting to know that almost none of commercial leaf wetness sensors come with an embedded temperature sensor, which begs the question how do they then compensate for the effect of temperature? The answer is "they don't". This takes us to the issue of unreliability of leaf wetness sensors that most often resemble leaves, but don't function as one and can't detect leaf wetness accurately enough.
My academic research at the university and later on extensive R&D at DurUntash Lab have shown that compensating leaf wetness sensor output for temperature change can significantly increase the accuracy of determining wetness onset and duration. A leaf wetness sensor with temp-compensated output can be used as a reliable tool in research and automatic systems for fruit and crop loss management.
INSHU LWS Sensor
Measurement principle
The INSHU LWS sensor mimics plant leaves and can be used to measure leaf temperature, wetness onset, and wetness duration. It can even quantify the amount of moisture on the surface of the sensor. The INSHU leaf wetness sensor falls into the category of flat dielectric leaf wetness sensors. It does not require any painting, preparation or calibration and all sensors are the same (high sensor uniformity) in terms of wetness readings under the same conditions. The sensor can sense the tiniest amount of water (condensed or frozen), not only on the surface of the sensor, but anywhere in its sensing sphere (a few cm from the upper surface; detects fog). This is because the sensor behaves like an RF antenna and creates an electromagnetic field around itself to measure the dielectric permittivity of water or ice. This measurement is later calibrated and related to the surface wetness.
Considering its importance, the INSHU LWS sensor also measures temperature. DurUntash Lab readers and dataloggers use an algorithm that takes Ta and raw wetness readings and delivers leaf wetness measurements that are reliably independent of changes in air temperature. The temperature compensation means sensor readings are accurate enough to not only tell there is water or ice on the surface of the sensor, but how much. The high level of accuracy makes the INSHU LWS perfect for automation projects.
Data interpretation
DurUntash Lab readers and data loggers report three numbers with each sensor measurement: 1) probe temperature, 2) leaf wetness (LW; scaled to -3 to 35%), and 3) raw wetness measurement (16-bit resolution). Depending on the installation method and other factors the readings might exceed 35%, be less than -3%, or never reach 35%. Any value between -3 and 0 is considered noise. A value that is above zero (0) indicates leaf wetness onset, and the higher the leaf wetness values the higher the amount of moisture on the surface of the sensor. Leaf wetness duration is the time period that above-zero values are recorded. Example real-time temperature and wetness data from an INSHU LWS sensor are plotted in Fig. 3. The data were collected using the DurUntash Lab wireless monitoring system.
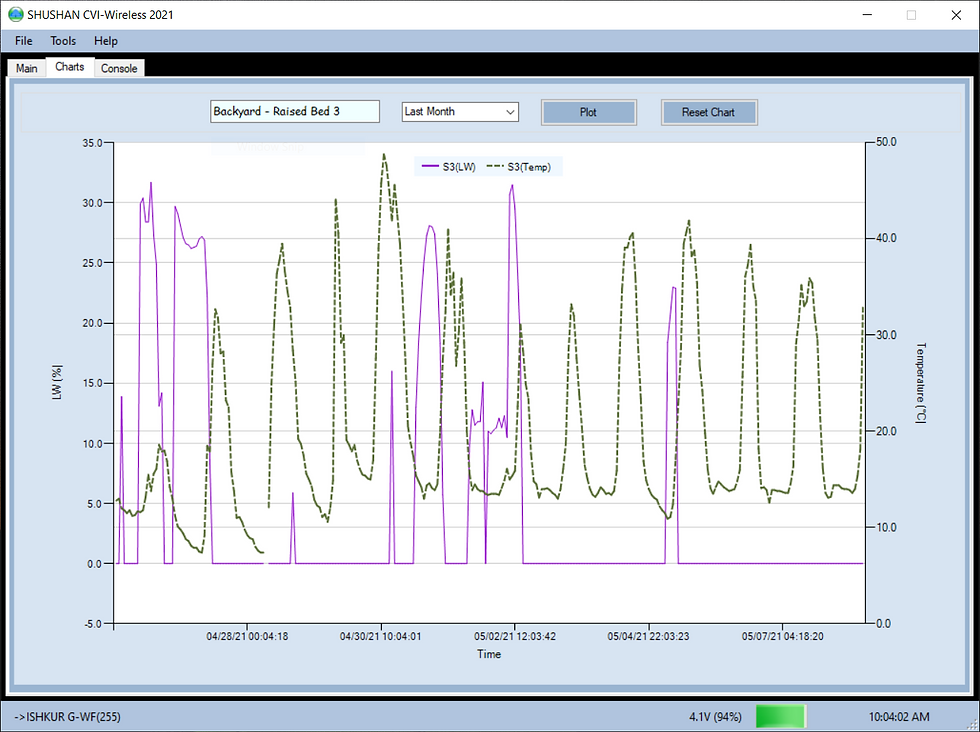
Temperature-compensation algorithm
To develop an automatic temperature-compensation algorithm, we installed INSHU LWS sensors in direct sunlight and studied the effect of temperature fluctuations on sensor readings. The algorithm takes temperature and wetness readings and delivers leaf wetness measurements that are reliably independent of changes in air temperature. This is unique to DurUntash Lab INSHU LWS sensor and all other similar sensors in the market provide leaf wetness readings that cannot be used without inspection and interpretation of data by the user (for consistency and temperature dependence).
As you can see in Fig. 3, even though temperature fluctuations exceeded 30°C on some days, the leaf wetness measurement are consistent and reliable. The sensor is very sensitive to moisture and can detect a single drop of water on the sensor surface (or even heavy fog in some cases). This amount of moisture is enough to increase the readings to values above 5%. Values below 0% that fluctuate are not an indication of wetness, but the result of noise and extremely high temperatures (> 40°C) (With the updated INSHU algorithm, noise is effectively filtered and therefore can no longer be seen).
We strongly recommend that you use the INSHU LWS sensor with one of our readout devices or dataloggers to unlock the full potential of the sensor. If you are using your own readout device or data acquisition systems, the sensor output will be temperature and raw voltage. With non-DurUntash data loggers, you need to convert raw voltage readings to leaf wetness, carry out temperature compensation, and establish a wetness threshold for your setup. Raw wetness readings depend on the readout device (custom-built electronics or compatible dataloggers) that the INSHU LWS is connected to and the resolution of the used analog to digital convertor. As an example, the ADS1115 ADC (16-bit resolution) will report numbers that may range from 10,000 to 15,000 when set for 2x gain.
INSHU LWS vs PHYTOS 31
Leaf wetness data collected using the INSHU LWS (DurUntash Lab, San Diego, CA) and PHYTOS 31 (Decagon / METER Group, Pullman, WA) sensors are depicted in Fig. 4 and Fig. 5, respectively. The INSHU LWS was installed in the field and captured both rainfall events and dew formations. The INSHU LWS embedded temperature sensor recorded a temp change of 8 to 41°C. The PHYTOS 31 was installed in a cherry orchard (in the canopy) where the temperature fluctuated from 24 to 31°C during our experiments. The PHYTOS 31 does not provide temperature output so we used a microclimate unit to measure air temperature (Osroosh and Peters, 2019).
As it can be seen in the graph, the INSHU LWS sensor leaf wetness baseline is a straight line (zero). The PHYTOS 31, on the other hand, has trouble distinguishing between the ambient temperature change, noise and leaf wetness. As a result, the PHYTOS 31 generates many false positive signals detecting leaf wetness when no moisture is present on the sensor surface.
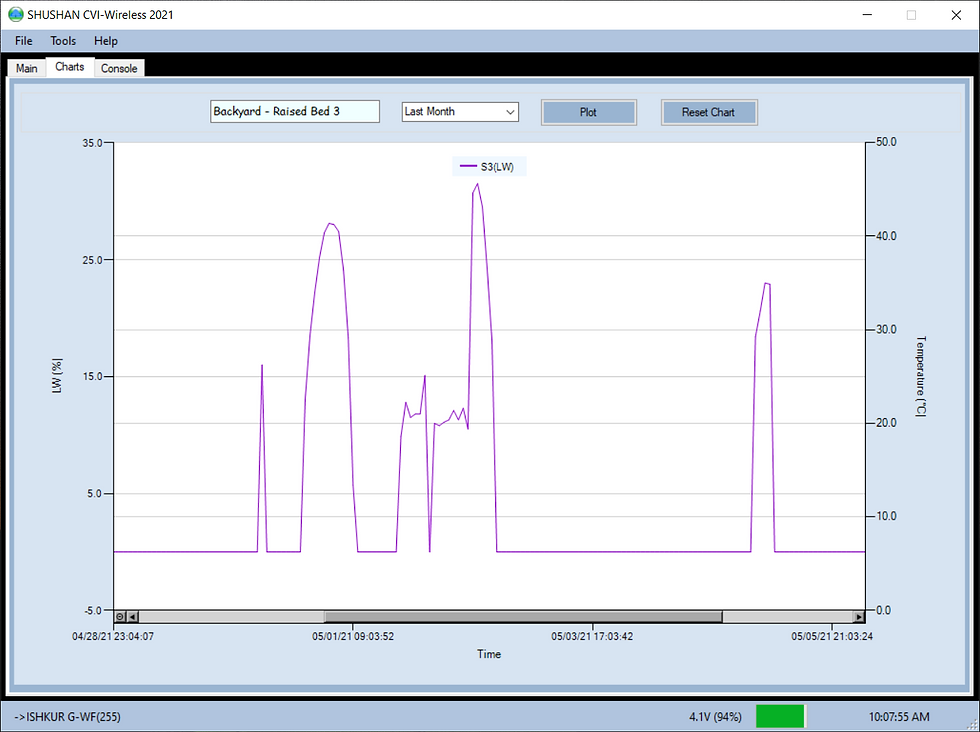
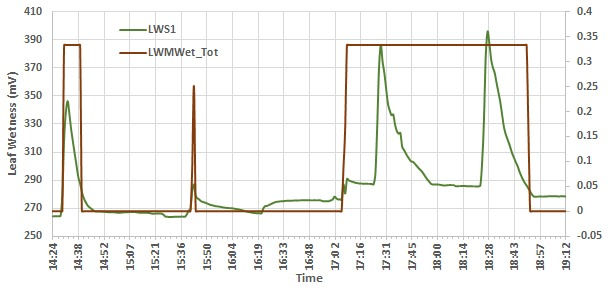
Conclusion
Leaf wetness and temperature are very important parameters in fruit and crop loss management. These parameters can be measured using so called "leaf wetness sensors", which can be categorized into dielectric and resistant type sensor. The problem is that most commercially available leaf wetness sensors do not provide reliable measurements. The key issue with these sensors is their temperature dependence. There is also no standardized method for the calculation of leaf wetness onset and duration using resistance-type leaf wetness sensors.
All things considered, I believe a "reliable" leaf wetness sensor
should be dielectric (capacitive),
comes pre-coated,
provides ambient temperature as an output (used for wetness temp-compensation) or provides temp-compensated wetness readings, and
mimics plant leaves in terms of properties (difficult to achieve).
The INSHU LWS sensor from DurUntash Lab is the only commercial sensor that meets all these criteria.
References
Osroosh, Y., Peters, R.T., 2019. Detecting fruit surface wetness using a custom-built low-resolution thermal-RGB imager. Computers and Electronics in Agriculture, 157: 509-517.
Sentelhas, P.C., Gillespie, T.J., Santos, E.A., 2007. Leaf wetness duration measurement: comparison of cylindrical and flat plate sensors under different field conditions. International Journal of Biometeorology, 51: 265–273.
Comentarios